Spending too much on big data? Try small data and master data management first: Together, small data and master data management reveal new insights into your business
Over the past few years working in data governance, I have encountered many projects aiming to re-write decision making processes using ”big data.” Data lakes, predictive analytics, AI and a cacophony of other acronyms have for many people veiled the complexity of big data, which pledged to deliver the four golden eggs of data insight; 1) better decision making, 2) happier customers, 3) freedom from risk and 4) identification of new opportunities.
I have much sympathy for the managers I have seen, left to contemplate their befogged bar charts, delivered from obscure data sources and massaged into place by employees with futuristic sounding job titles, unaware of their existence until last week.
I can hear the questions from the management team that follow. “I can see a kind of pattern, but what decision do we need to make? Why is this happening? How sure are we about the trend and the cause?”
An uncomfortable silence ensues.
A recent report by BearingPoint highlighted this challenge, observing that “the heads of big companies act more on gut feel than on hard data.” That is a situation any informed business can no longer find acceptable.
Big data vs. small data: The importance of finding cause in data
While it is true, thanks to big data, voluminous sources of seemingly disparate information can now be combined, analyzed and correlated to identify trends and patterns, it does not necessarily follow, that such analysis can explain causes. For example, a trend in customers cancelling contracts does not necessarily mean they are all unhappy. Indeed, how do you guard against bias in your trend analysis in the first place?
So why is it important for you to understand cause? Quite simply, because the data behind cause weighs heavily on data-driven decision making. As correlation does not imply causation, there is significant scope for misjudgment using trend analysis alone.
Big data definition: Finding patterns and inferring correlations in large data sets often constitutes goals of big data projects. The data can be largely transactional, historical, of dubious quality and without common (metadata) definition. AI and machine learning initiatives will, nevertheless, use such sources to improve analytics and learn how to automate processes; the goal being to accelerate the data-to-decision process.
Those suffering most in adapting to new decision-making processes are often more traditional companies whose leaders are still unversed in data culture and its potential impact on business transformation. Their experience, bias and unquestioning belief in the accuracy of the data presented, forms the basis of their sedate decision-making. They witness, in contrast, the arrival of new and disruptive competitors whose data-centric mindset is intrinsic to their culture and vision, making bold yet informed decisions at speed, seeing cause through the lens of “small” data.
Small data definition: Small data refers to reduced data sets that are understandable, often simple and collected dynamically. They often don’t need vast computing resources to collect and analyze. Small data can be pertinent and insightful on its own and relate to a particular event, for example, recording the customer’s sentiment at a moment in time.
What is happening now? No… right now!
As anyone who manages data can attest, volume and quality are two forces that don’t always go hand-in-hand. As data has gotten ever bigger, the ability to manage and derive value and insight from small data has become increasingly consequential. More digital connections are being made in real-time. Customer attention spans are shrinking, while windows of opportunity are closing faster. It’s an environment that demands faster decision-making, which allows for less time to curate and present the data that supports it.
Small data provides discernible signals to assist in this type of accelerated decision environment. Small data is able to tell you what is happening right here, right now. What is this machine currently doing? Where is that package located? What type of sentiment is your customer expressing?
Small data and multidomain master data management go hand-in-hand
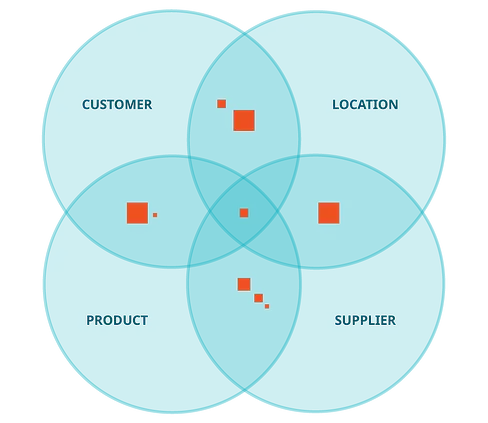
When it comes to data-driven decision making, multidomain master data management is the ideal home to create insight and value from small data. Here’s why.
-
Decision making at the edge needs governed master data
Trustworthy decisions need accurate, transparent data, along with the necessary governance to make them informed and auditable
-
Cause analysis needs master data
Small data describing the context of what is happening now, coupled with its corresponding master data, forms the basis for making better, data-driven decisions
-
Master data management improves trend and correlation outcomes
The resulting combination of master data and contextually small data can be fed back into big data repositories, resulting in improved trend analysis
-
Correlated data must be accurate and unbiased to support cause detection
Master data management provides the governance mechanism for the required transparency of both master data and the accompanying small data
-
Small data benefits from multidomain master data management
Decisions supported by small data often find themselves at the intersection of multiple domains; for example, the customer (master data), their location (small data), the product (master data), their current basket contents (small and master) and the weather (small). Master data management provides an ideal mechanism to unite this data at a moment in time while keeping it under control with data governance policy rules.
-
Cause needs to be shared
For action to be taken based on small data, it needs to be governed and distributed almost immediately. Master data management was designed for this very purpose.
Ultimately, it is the ability of master data management to support the accuracy of cause determination that makes it so fit for this purpose, while also supporting the auditability of any decisions taken related to the cause. Managing small data with master data management is part of an augmented master data management strategy, whereby additional business value is developed via the bias of having trustworthy master data.
Small data and multidomain master data management in action
Small data and multidomain master data management are helping to make a big impact in very diverse applications. Here are some examples.
Employee retention
Detection of small changes in the ways workplace collaborative tools are used, such as meeting agenda changes and numbers of messages exchanged, can capture levels of motivation and help to establish retention strategies.
• Master data domains: employee, location, organization, role
• Small data: collaboration tool, usage frequency, date, duration
Personalized shopping
New levels of personalized shopping will be enabled using a small data approach coupled with master data management.
The combination of wearable and consumer held devices, sensors on shelves, surveillance cameras and tagged products has the potential to produce highly valuable small data sets that can drive improvement strategies in the customer’s in-store experience.
• Master data domains: customer, product, location
• Small data: Location, time, sensor data
Customer service
When a customer contacts a helpdesk, recognition of the phone number can accelerate handing of the enquiry. For example, knowing that a passenger’s flight is delayed, their call is likely to be related and automated call answering can respond accordingly.
• Master data domains: customer, product
• Small data: phone/device, location, service in progress
A small data approach can have a significant impact on the ability to take fast, data-driven decisions. Multidomain master data management provides the ideal place to host your small data against the key domains with which it is associated – customer, product location, etc. multidomain master data management provides the governance capabilities needed to ensure that data-driven decisions are based on reliable, auditable and transparent data.