The language of master data management (MDM) is packed with definitions, acronyms and abbreviations used by analysts, data scientists, CDOs and IT managers. This dictionary offers simple explanations of the most common MDM terms and helps you navigate the MDM landscape.
A | B | C | D | E | F | G | H | I | J | K | L | M | N | O | P | Q | R | S | T | U | V | W | X | Y | Z
Application Data Management - ADM
ADM is the management and governance of the application data required to operate a specific business application, such as your ERP, CRM or supply chain management (SCM). Your ERP system’s application data is data associated with products and customers. This may be shared with your CRM, but in general, application data is shared with only few other applications and not widely across the enterprise.
ADM performs a similar role to MDM, but on a much smaller scale as it only enables the management of data used by a single application. In most cases, the ADM governance capabilities are incorporated into the applications, but there are specific ADM vendors that provide a full set of capabilities.
See also: Master Data
More information:
Application Programming Interface - API
An API is an integrated code of most applications and operating systems that allows one piece of software to interact with other types of software. In master data management, not all functions can necessarily be handled in the MDM platform itself. For instance, you want to be able to deliver product data from your MDM to the ecommerce system or validate customer data using a third-party service. The API makes sure your request is delivered and returns the response.
The use of APIs is a core capability of the MDM enterprise platform. As the single source of truth, the MDM needs to connect with a wide range of business systems across the enterprise.
See also: Connector, DaaS, Data Integration
More information:
Architecture
An MDM solution is not just something you buy, then start to use. It needs to be fitted into your specific enterprise setup and integrated with the overall enterprise architecture and infrastructure, which is why MDM architecture is required as one of the first steps in an MDM process. Your MDM strategy should include a map of your data flows and processes, which systems are delivering source data, and which rely on receiving accurate data to operate. This map will show where the MDM fits into the enterprise architecture.
See also: Implementation Styles
More information:
- STEP – Stibo Systems Enterprise Platform
- How to Build Your Master Data Management Strategy in 7 Steps
- MDM Academy course: MDM Solution Architecture
Artificial Intelligence - AI
In the MDM universe, AI is relevant in two ways. First, AI is an integral part of the MDM that uses AI and the underlying machine learning technology to classify data objects, such as products. AI searches for certain identifiers to find patterns in fragments of information, e.g., a few attributes or a picture of a product and makes sure the product is classified correctly in the product taxonomy. This capability is used in product onboarding. If the object does not match the classification criteria it can be sent to a clerical review which will help train the AI. Second, AI also relies on MDM when used in external applications. AI needs to know which identifiers to search for. These identifiers are described and governed in the MDM data model. MDM ensures the results of AI processing are trustworthy.
See also: Machine Learning, Auto-Classification
More information:
- AI & ML - So, What's All the Hype About?
- Advance Your AI Agenda with Master Data Management Extract value from AI and machine learning faster with the power of MDM
Asset Data
Enterprise assets can be physical (equipment, buildings, vehicles, infrastructure) and non-physical (data, images, intellectual property). In any case, it’s a subject that is owned by a company. Assets have data, such as specifications, location, bill-of-materials and unique ID. This data can be leveraged in different ways and needs to be managed, for instance for preventative maintenance. An MDM system can help to describe how assets relate functionally, perform and configure, essentially holding the digital twin of an asset. Having reliable information on your assets, can answer important questions like “What is the current condition of our assets that are supporting this business process? Who is using them? Where are they located?” Please note this important distinction: Asset data is your assets’ master data whereas data that is generated by your assets is called sensor data, time series data or IoT data. Master data has the quality of being low-volatile whereas IoT data is constantly changing and increasing. Asset data represents what you know with certainty about your assets.
See also: DAM, Digital Twin
More information:
Attributes
In MDM, an attribute is a specification or characteristic that helps define an entity. For instance, a product can have several attributes, such as color, material, size, ingredients or components. Typical customer attributes are name, address, email and phone number. MDM supports the management and governance of attributes to provide accurate product descriptions, identify customers and create golden records. Having accurate and rich attributes is hugely important for the customer experience as well as for the data exchange with partners and authorities.
See also: Data Entity, Data Object, Data Governance, Golden Record
More information:
Augmented MDM
Augmented master data management applies machine learning (ML) and artificial intelligence (AI) to the management of master data. Leveraging AI techniques can enhance data management capabilities to complement human intelligence to optimize learning and decision making. Augmented MDM enables companies to refine master data to optimize their business operations to run more efficiently and transform their businesses to drive growth.
See also: AI
More information:
Auto-Classification
Auto-classification, computer-assisted classification or machine-supervised classification, is a method of automatically categorizing items into predefined categories, classes and industry standards using machine learning algorithms. It can be used as a part of a product information management (PIM) system to automatically categorize and organize product information into different product categories and attributes, for example, when products are onboarded with a minimal set of data, such as brand name and item number. Auto-classification, and computer assisted classification, speed up supplier item onboarding and leads to an up-front more correct classification of items, leading to better data quality through a correct use of templates of the item records. Predictions for classifications are associated with a confidence range and if the confidence of the prediction falls below a set threshold, the product is sent to clerical review for manual classification, which in turn can be used to re-train the classification algorithm.
More information:
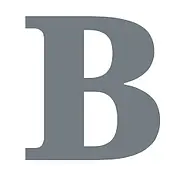
Big Data
Big data is characterized by the three Vs: Volume (a lot of data), Velocity (data created with high speed) and Variety (data comes in many forms and ranges). Big data does not only exist on the internet but within single companies and organizations who need to capitalize on that data.
Finding patterns and inferring correlations in large data sets often constitutes goals of big data projects. The purpose of using big data technologies is to capture the data and turn it into actionable insights. The information gathered from big data analytics can be linked to your master data and thereby provide new levels of insights.
See also: Data Analytics
More information:
Bill of Materials - BOM
In manufacturing, a bill of materials is a list of the parts or components that are required to build a product. A BOM is similar to a formula or recipe, but whereas a recipe contains both the ingredients and the manufacturing process, the BOM only contains the needed materials and items. Manufacturers rely on different digital BOM management solutions to keep track of part numbers, unit of measure, cost and descriptions.
See also: Attributes
Business Intelligence - BI
Business intelligence is a type of analytics. It analyzes the data that is generated and used by a company in order to find opportunities for optimization and cost cutting. It entails strategies and technologies that help organizations understand their operations, customers, financials, product performance and many other key business measurements. Master data management supports BI efforts by feeding the BI solution with clean and accurate master data.
See also: Data Analytics, Data Governance
Business Rules
Business rules are the conditions or actions that modify data and data processes according to your data policies. You can define numerous business rules in your MDM to determine how your data is organized, categorized, enriched, managed and approved. Business rules are typically used in workflows, e.g., for the validation of data in connection with import or publishing. As such, business rules are a vital tool for your data governance and for the execution of your data strategy as they ensure the data quality and the outcome you want to achieve.
See also: Workflow
More information:
Centralized Style
The centralized implementation style refers to a certain system architecture in which the MDM system uses data governance algorithms to cleanse and enhance master data and then pushes data back to its respective source system. This way, data is always accurate and complete where it is being used. The Centralized style also allows you to create master data making your MDM the system of origin of information. This means you can centralize data creation functions for supplier, customer and product domains in a distributed environment by creating the entity in MDM and enriching it through external data sources or internal enrichment.
See also: Implementation styles, Coexistence style, Registry style, Consolidation style
More information:
Change Management
Change management is the preparation and education of individuals, teams and organizations in making organizational change. Change management is not specific to implementing a master data management solution, it is, however, a necessity in any MDM implementation if you want to maximize the ROI. Implementing MDM is not a technology fix but just as much about changing processes and mindsets. As MDM aims to break down data silos, it will inevitably raise questions about data ownership and departmental accountabilities.
More information:
Cloud MDM
A hosted cloud MDM solution is being run on third-party servers. This means organizations don’t need to install, configure, maintain and host the hardware and software, which is outsourced and offered as a subscription service. Cloud MDM holds many operational advantages, such as elastic scalability, automated backup and around-the-clock monitoring. Most companies choose a hosted cloud MDM solution vs. an on-premises solution or choose to migrate their solution to the cloud.
See also: SaaS
More information:
Coexistence Style
The coexistence implementation style refers to a system architecture in which your master data is stored in the central MDM system and updated in its source systems. This way, data is maintained in source systems and then synchronized with the MDM hub, so data can coexist harmoniously and still offer a single version of the truth. The benefit of this architecture is that master data can be maintained without re-engineering existing business systems.
See also: Implementation styles, Centralized style, Registry style, Consolidation style
More information:
Connector
Connectors are application-independent programs that perform transfer of data or messages between business systems or external sources and applications, such as connecting your MDM platform to your ERP, analytics or to data validation sources or marketplaces. Connectors are a vital architectural component to centralize data and automate data exchange.
See also: API
More information:
Consolidation Style
The consolidation style refers to a system architecture in which master data is consolidated from multiple sources in the master data management hub to create a single version of truth, also known as the golden record.
See also: Implementation styles, Centralized style, Registry style, Coexistence style
More information:
Contextual Master Data
Contextual (or situational) master data management refers to the management of changeable master data as opposed to traditional, more static, master data. As products and services get more complex and personalized, so does the data, making the management of it equally complex. The dynamic and contextual MDM takes into consideration that the master data, required to support some real-world scenarios, changes. For example, certain personalized products can only be created and modeled in conjunction with specific customer information.
More information:
Customer Data Integration - CDI
CDI is the process of combining customer information acquired from internal and external sources to generate a consolidated customer view, also known as the golden record or a customer 360° view. Integration of customer data is the main purpose of a Customer MDM solution that acquires and manages customer data in order to share it with all business systems that need accurate customer data, such as the CRM or CDP.
See also: Customer MDM
More information:
Customer Data Platform - CDP
A customer data platform is a marketing system that unifies a company’s customer data from marketing and other channels to optimize the timing and targeting of messages and offers. A Customer MDM platform can support a CDP by ensuring the customer data that is consumed by the CDP is updated and accurate and by uniquely identifying customers. The CDP can manage large quantities of customer data while the MDM ensures the quality of that data. By linking the CDP data to other master data, such as product and supplier data, the MDM can maximize the potential of the data.
See also: CRM, Customer MDM
More information:
Customer MDM
Customer master data management is the governance of customer data to ensure unique identification of one customer as distinct from another. The aim is to get one single and accurate set of data on each of your business customers, the so-called 360° customer view, across systems and locations in order to get a better understanding of your customers. Customer MDM is indispensable for all systems that need high-quality customer data to perform, such as CRM or CDP. Customer MDM is also vital for compliance with data privacy regulations.
See also: CRM, CDP, GDPR
More information:
- The 26 Questions to Ask Before Building Your Business Case for Customer Data Transparency
- Dealing with GDPR
Customer Relationship Management - CRM
CRM is a system that can help businesses manage customer and business relationships and the data and information associated with them. For smaller businesses a CRM system can be enough to manage the complexity of customer data, but in many cases organizations have several CRM systems used to various degrees and with various purposes. For instance, the sales and marketing organization will often use one system, the financial department another, and perhaps procurement a third. MDM can provide the critical link between these systems. It does not replace CRM systems but supports and optimizes the use of them.
See also: ERP, Customer MDM
More information:
Data Analytics
Data analytics is the discovery of meaningful patterns in data. For businesses, analytics are used to gain insight and thereby optimize processes and business strategies. MDM can support analytics by providing organized master data as the basis of the analysis or link trusted master data to new types of information output from analytics.
More information:
- The Role of MDM as a Catalyst for Making Sense of Big Data
- Embedded Analytics Platform for MDM Solutions
Data as a Service - DaaS
Data as a Service is a cloud-based data distribution service focused on the real-time delivery of data at scale to high-volume data-consuming applications. As part of MDM, DaaS delivers a near real-time version of master data through a configurable API and serverless architecture. This removes the need to maintain several API services as well as the need to create multiple copies of data for each application.
See also: SaaS
More information:
- Data as a Service
- Master Data Management with a DaaS Extension for Data Consumption at High Scale
- Making Master Data Accessible: Wha is Data as a Service?
Data Augmentation
Data augmentation is a technique used in data science and machine learning to increase the size and diversity of a data set by creating new samples from existing data through modifications or transformations. The goal of data augmentation is to enhance the quality and robustness of the data and improve the accuracy and generalization of machine learning models. Master data augmentation refers to the process of enriching or expanding the existing master data of an organization by integrating new or external data sources. This can involve adding new attributes or fields to the existing master data, as well as updating or enriching the existing attributes with additional information. The goal is to improve the accuracy, completeness and relevance of the master data to enable better decision-making and execution of business processes. By incorporating new data sources and expanding the scope of the master data, organizations can gain a more comprehensive view of their operations and assets.
See also: Augmented MDM
More information:
Data Catalog
A data catalog is a tool that provides an organized collection of metadata, descriptions and information about the data assets available within an organization. A data asset can refer to any type of structured or unstructured data that is generated, collected or maintained by an organization. The purpose of a data catalog is to make it easier for users to discover, understand and access data assets. The catalog typically provides a searchable and browsable interface that allows users to find and explore data assets based on various attributes, such as name, type, format, owner, and usage. Master data management (MDM) and data catalogs are complementary technologies that can work together to improve data management practices. MDM provides a centralized repository for managing the most important data assets, such as customer and product data, while a data catalog provides an interface for discovering and accessing data assets across the organization.
Data Cleansing
Data cleansing is the process of identifying, removing and correcting inaccurate data records, for example by deduplicating data. Data cleansing eliminates the problems of useless data to ensure quality and consistency throughout the enterprise and is an integral and basic process of master data management. Clean data is essential for a company’s ability to make data-driven decisions, as well as for delivering great customer experiences.
See also: Deduplication
Data Democratization
Democratizing data means to make data available to all layers of an organization and its business ecosystem, across departmental boundaries. Hence, the opposite of data democratization is to store data in silos managed and controlled by a single department without visibility for others. Data democratization is only possible if data is transparent. That includes its quality and sources, how it is shared and used, and who is accountable for the data quality as well as for the interpretation of the data.
See also: Data silo, data transparency
More information:
Data Domain
In master data management, a data domain refers to a specific set or category of data that share common characteristics, such as data type, purpose, source, format, or usage. Data domains are often used to organize and categorize data assets within an organization, and they play a critical role in data governance and data quality management. For example, the customer data domain includes data such as name, address, email, phone number and demographic information. Another example could be product data, including attributes such as size, color, function, technical specifications and item number. Different domains can be governed in conjunction and thus provide new valuable insights, such as where and how a product is used. By defining data domains and their attributes, organizations can establish a common understanding of their data and ensure consistency, accuracy, and completeness across different systems and applications. This, in turn, can help organizations make better data-driven decisions, improve operational efficiency, and comply with regulatory requirements.
See also: Multidomain, Data governance, MDM, PIM, Zones of Insight
More information:
Data Enrichment
Data enrichment refers to the process of enhancing, refining or improving existing data sets by adding new information, attributes or context to them. The goal of data enrichment is to increase the value, relevance and accuracy of the data for analysis, decision-making and improving customer experiences. Product data enrichment is particularly important for providing good customer experiences and preventing product returns. Customer data enrichment can help companies gain a more comprehensive view of their customers. Data enrichment can involve various techniques and sources, such as: - Data augmentation: adding new data points or fields to existing data sets, such as demographic, geographic, behavioral or transactional data. - Data integration: combining data from multiple sources or systems to create a unified view of the data.
More information:
Data Fabric
Data fabric is a data architecture that connects and enhances your operating systems to provide these systems with clean, real-time data at scale. It’s an integrated layer on top of systems that these systems can subscribe to. It is designed to provide a unified view of data that can be accessed and used by different applications and users within an organization. Data fabric improves collaboration through a common shared data language and democratization of data. A multidomain master data management platform can facilitate a data fabric through integration of data sources and built-in data governance. However, data fabric extends beyond the capabilities of a single technology. It typically includes a variety of technologies and components, including data virtualization engines, data integration tools, metadata management systems and data governance policies. These components work together to provide a comprehensive view of data that can be accessed and used by different stakeholders. One of the key benefits of a data fabric is that it enables organizations to access and analyze data in real-time, regardless of where the data is located or how it is stored.
Data Governance
Data governance is a collection of practices and processes aiming to create and maintain a healthy organizational data framework. It is a set of policies, procedures and guidelines that are put in place to ensure that data is accurate, consistent and compliant with regulations and standards. Data governance can include creating policies and processes around version control, approvals, etc., to maintain the accuracy and accountability of the organizational information. Data governance is as such not a technical discipline but a discipline to ensure data is fit for purpose. Data governance includes a variety of activities, such as data quality management, data security, data privacy, data classification, data lifecycle management and data stewardship. These activities are designed to ensure that data is properly managed and used throughout its lifecycle, from creation to deletion. Data governance is typically led by a data governance team or committee that is responsible for creating and enforcing data policies and standards. This team may include representatives from various departments within an organization, such as IT, legal, compliance and business operations. Data governance can be supported by master data management capabilities that are configurable to execute data policies.
More information:
- What Is Master Data Governance – And Why You Need It?
- How to Develop Clear Data Governance Policies and Processes for Your MDM Implementation
- Ten Useful Steps to Master Data Governance
Data Hierarchy
In the context of master data management (MDM), data hierarchy refers to the organization and classification of data elements based on their level of importance and their relationships to each other. At the top of the data hierarchy are the master data domains. These are the core data entities, such as customers, products, suppliers and employees. Beneath the master data domain, e.g., product, are the different product types and classes. Under these you will find the stock keeping units (SKU) and their attributes. At the bottom of the data hierarchy is the reference data. Reference data includes categories like product classifications, industry codes and currency codes. Master data management helps organize data into logical hierarchies which is essential to become a data-driven organization.
See also: Data Modeling, Data Domain, Reference Data
Data Hub
A data hub, or an enterprise data hub (EDH), is a database which is populated with data from one or more sources and from which data is taken to one or more destinations. A master data management system is an example of a data hub, and therefore sometimes goes under the name master data management hub.
More information:
- Stibo Systems Enterprise Platform (STEP)
- Deliver Insight to the Edge of the Enterprise with a Multidomain MDM-Powered Digital Business Hub
Data Integration
Data integration is the process of combining data from different sources into a unified view. It involves transforming and consolidating data from various sources such as databases, cloud-based applications and APIs into a single, coherent dataset. One of the biggest advantages of a master data management solution is its ability to integrate with various systems and link all of the data held in each of them to each other. A system integrator will often be brought on board to provide the implementation services.
See also: API
More information:
Data Lake
A data lake is a central place to store your data, usually in its raw form without changing it. The idea of the data lake is to provide a place for the unaltered data in its native format until it’s needed. Data lakes allow organizations to store both structured and unstructured data in a single location, such as sensor data, social media data, weblogs and more. The data can be stored in different formats. Data lakes are highly scalable, allowing organizations to store and process vast amounts of data as needed, without worrying about storage or processing limitations. Certain business disciplines, such as advanced analytics, depend on detailed source data. However, since the data in a data lake has not been curated and derives from many different sources, this introduces an element of risk when the ambition is data-driven decision making. Data that is stored in a data lake is not reconciled and validated and may therefore contain duplicate or inaccurate information. Applying master data management can improve the accuracy and help identify relationships and thus enhance the transparency.
See also: Data Warehouse
Data Maintenance
In order for any data management investment to continue delivering value, you need to maintain every aspect of a data record, including hierarchy, structure, validations, approvals and versioning, as well as master data attributes, descriptions, documentation and other related data components. Maintenance is often enabled by automated workflows, pushing out notifications to, e.g., data stewards when there’s a need for a manual action. Maintenance is an important and ongoing process of any MDM implementation.
Data Mesh
Data mesh is an approach to data architecture that emphasizes the decentralization and democratization of data ownership and governance. It aims to address some of the common challenges of traditional centralized data architectures, such as slow time-to-market, siloed data and lack of agility. Traditional data storage systems can continue to exist but instead of a centralized data team that owns and governs all data, data mesh advocates for data ownership to be distributed among cross-functional teams that produce and consume data. This federated system helps eliminate many operational bottlenecks. The key idea behind data mesh is to treat data as a product and apply product thinking principles to data management. This implies clear product ownership, product roadmaps and product metrics. Data products should be designed to meet the specific needs of consumers, and should be evaluated based on their value to the business. While data mesh and master data management have different approaches to data management, they can complement each other in a hybrid data management approach. For example, domain-specific teams in a data mesh architecture can use MDM to ensure that their data products are consistent with enterprise-wide master data standards. In this way, data mesh and MDM can work together to ensure that data is accurate, consistent, and trustworthy across the enterprise.
See also: MDM
Data Modeling
Data modeling is the process of creating a conceptual or logical representation of data objects, their relationships and rules. It is used to define the structure, constraints and organization of data in a way that enables efficient data management and analysis, as well as supports your business model. Data modeling is an essential component of master data management (MDM) as it provides a principle for organizing and managing master data. In MDM, data modeling is used to define the attributes, relationships and hierarchies of master data entities such as customers, products and locations. It also helps in identifying and resolving data conflicts and duplicates by establishing unique identifiers and rules for data matching and merging. Data modeling in master data management is a process in the beginning of an MDM implementation where you accurately map and define the relationship between the core enterprise entities, for instance your products and their attributes. Based on that you create the optimal master data model that best fits your organizational setup.
Data Monetization
Data monetization is generally understood as the “process of using data to obtain quantifiable economic benefit” (Gartner Glossary, Data Monetization). There are three typical ways to achieve this:
- Using data to make better decisions and thus enhance your business performance
- Sharing data with business partners for mutual benefit
- Selling data as a product or service
Whichever way you choose, data monetization gets more profitable if you can provide context to the data and make it insightful. The more insight your data provides, the more valuable it is. Multidomain master data management can help add context and that way increase the value of your data.
More information:
Data Object
The data object is what you aim to enrich and identify uniquely using master data management (MDM). A data object has a unique ID and a number of attributes that it may share with other data objects, such as a specific customer that may share the address with another specific customer. Products, suppliers, customers and locations are examples of types of data objects in a master data management context. They are organized in data models that can contain relations between data objects. A multidomain MDM can show relations across data object types.
See also: Data Modeling
Data Onboarding
Data onboarding is the process of transferring data from an external source into your system, e.g., product data from suppliers or content service providers to your PIM system or from an offline database to an online environment. The onboarding process can be be handled by data integration via an API, a data onboarding tool or by importing xml files. The speed of data onboarding can be crucial for reducing your time to market. Sophisticated onboarding processes can help maintain data quality by flagging duplicates or incomplete data. If your onboarding tool is supported by AI, it may suffice to onboard fragments of information, such as an item number or GTIN number and a brand name.
Data Policy
Your data policy is a set of rules and guidelines for your data management to ensure data quality as well as data processes are aligned with business goals. Your data policy defines data ownerships, stewardships, how to store and share data. A thorough data policy should be in place prior to any system implementation in order to ensure accountability and that data is clean and fit for purpose.
Data Pool
A data pool is a repository of data where trading partners can maintain and exchange information about products in a standard format. Suppliers can, for instance, export data to a data pool that cooperating retailers can then import to their ecommerce system. Data pools, such as 1WorldSync and GS1, enable business partners to synchronize data and reduce their time to market. An MDM system that supports various data pool formats can facilitate the data exchange and minimize the manual work needed.
See also: GS1
More information:
- Connect your applications to take your data further, faster
- Achieve Effortless Compliance with Industry Standards
Data Quality
Data quality, or just DQ, refers to the overall accuracy, completeness, consistency, timeliness and relevance of data. The quality of data can have a significant impact on the accuracy and effectiveness of decision-making processes, as well as on the success of business operations that rely on data.
The quality of data is therefore of particular interest for data analysts and business intelligence. Accuracy refers to the extent to which data reflects reality, and it involves ensuring that the data is correct and free from errors. Completeness refers to the extent to which all necessary data is available, and there are no missing values. Consistency refers to the extent to which data is uniform and follows predefined standards, so it can be compared or analyzed. Timeliness refers to the extent to which data is available when it is needed and reflects the current state of affairs. Finally, relevance refers to the extent to which data is useful and applicable to a specific task or purpose.
100% data accuracy is in most cases not attainable, and also not desirable. Most organizations aim for the data quality that is 'fit for purpose'. The finest job for master data management is to ensure the quality of master data to enable qualified decision making.
Data Silo
The term data silo describes when crucial data or information — such as master data — is stored and managed separately and isolated by individuals, departments, regions or systems. The siloed approach results in data being not easily accessible or shared with other parts of the organization. In other words, data silos are a barrier that prevents the free flow of information within an organization. Data silos are often created unintentionally as different departments adopt different software systems or databases to manage their data. These silos can make it difficult for other departments to access important information, which can lead to redundant efforts, inconsistencies and inaccurate or incomplete data analysis. Master data management can help mitigating the negative impact of data silos by way of integration with business applications, such as ERPs and CRMs, thus providing a single source of truth.
More information:
- Data Silos Are Problematic. Here's How You Turn them into Zones of Insight
- Connecting Siloed Data with Master Data Management
Data Swamp
A data swamp occurs where a large volume of data is collected and stored without proper organization, management or governance. It is a data repository that is poorly managed and lacks structure, making it difficult or impossible to use for analysis or decision-making. The cause of a data swamp is when organizations collect data without a clear understanding of how it will be used or how it fits into the organization's broader goals and objectives. The consequences of a data swamp can include wasted resources, reduced productivity and even regulatory issues as data that is poorly managed may be subject to compliance violations or data breaches. To prevent a data swamp, organizations must adopt a data management strategy that includes proper data governance, metadata management and data quality controls. The capabilities of master data management support streamlining of data to make it trustworthy and actionable.
See also: Data Lake
Data Synchronization
Synchronization of master data is the process of ensuring that data is consistent and up-to-date across multiple systems or devices. Master data management ensures that all users have access to the most recent and accurate master data. Data synchronization is crucial in situations where multiple users or systems need to access and modify the same data, such as in a collaborative work environment or a mobile application that accesses data from a central server.
Data Syndication
Syndicating data is important for manufacturers and brand owners in order to share accurate, channel-optimized product data and content with retailers, data pools and marketplaces. Data syndication entails mapping, transforming and validating data. A master data management solution can automate the syndication process using built-in support for industrial classification standards or an integrated data syndication tool.
More information:
Data Transparency
Data transparency refers to the end-to-end insight into your most valuable data. It involves breaking down silos and barriers that obstruct the visibility, clarity and flow of trusted data. Data transparency includes knowledge about your data's completeness, where it comes from, who can access it, who is accountable for it, etc. Having data transparency enables you to comply with data regulations and standards and make better decisions based on insight. This insight is particularly important in order to meet the growing sustainability demands.
More information:
- Unlock the Power of Your Data with a Transparent Approach to Master Data Management
- How Data Transparency Enables Sustainable Retailing
- Achieving Supply Chain Transparency with Supplier Data
Digital Asset Management - DAM
The business management of digital assets, most often images, videos, digital files and their metadata. Many businesses have a standalone or home-grown DAM solution, inhibiting the efficiency of the data flow and thereby delaying processes, such as on-boarding new products into an e-commerce site. MDM lets you handle your digital assets more efficiently and connects it to other data. DAM can be a prebuilt function in some MDM solutions.
Deduplication
The process of eliminating redundant data in a data set, by identifying and removing extra copies of the same data, leaving only one high-quality data set to be stored. Data duplicates are a common business problem, causing wasted resources and leading to bad customer experiences. When implementing a Master Data Management solution, thorough deduplication is a crucial part of the process.
Digital Transformation
(or Digital Disruption). Refers to the changes associated with the use of digital technology in all aspects of human society. For businesses, a central aspect of Digital Transformation is the "always-online" consumer, forcing organizations to change their business strategy and thinking in order to deliver excellent customer experiences. Digital Transformation also has major impact on efficiency and workflows (e.g., the so-called Fourth Industrial Revolution driven by automation and data, also known as Industry 4.0). MDM can play a crucial role in driving digital transformations, as the backbone of these are data.
Data Universal Numbering System (D-U-N-S)
A D-U-N-S number is a unique nine-digit identifier for each single business entity, provided by Dun & Bradstreet. The system is widely used as a standard business identifier. A decent MDM solution will be able to support the use of D-U-N-S by providing an integration between the two systems.
Enterprise Asset Management - EAM
The management of the assets of an organization (e.g., equipment and facilities).
Enterprise Resource Planning - ERP
Refers to enterprise systems and software used to manage day-to-day business activities, such as accounting, procurement, project management, inventory, sales, etc. Many businesses have several ERP systems, each managing data about products, locations or assets, for example. A comprehensive MDM solution complements an ERP by ensuring that the data from each of the data domains used by the ERP is accurate, up-to-date and synchronized across the multiple ERP instances.
Extract, Transform and Load - ETL
A process in data warehousing, responsible for pulling data out of source systems and placing it into a data warehouse.
Golden Record
In the MDM world, also sometimes referred to as "the single version of the truth." This is the state you want your master data to be in and what every MDM solution is working toward creating: the most pure, complete, trustable data record possible.
Global Standards One - GS1
The GS1 standards are unique identification codes used by more than one million companies worldwide. The standards aim to create a common foundation for businesses when identifying and sharing vital information about products, locations, assets and more. The most recognizable GS1 standards are the barcode and the radio-frequency identification (RFID) tags. An MDM solution will support and integrate the GS1 standards across industries.
Hierarchy Management
An essential aspect of MDM that allows users to productively manage complex hierarchies spread over one or more domains and change them into a formal structure that can be used throughout the enterprise. Products, customers and organizational structures are all examples of domains where a hierarchy structure can be beneficial (e.g., in defining the hierarchical structure of a household in relation to a customer data record).
Identity resolution
A data management process where an individual is identified from disparate data sets and databases to resolve their identity. This process relates to Customer Master Data Management.
Also see CMDM.
Information
Information is the output of data that has been analyzed and/or processed in any manner.
Also, see Data.
Internet of Things - IoT
Internet of Things is the network of physical devices embedded with connectivity technology that enables these "things" to connect and exchange data. IoT technology represents a huge opportunity—and challenge—for organizations across industries as they can access new levels of data. A Master Data Management solution supports IoT initiatives by, for example, linking trusted master data to IoT-generated data as well as supporting a data governance framework for IoT data.
Learn more about the link between IoT and MDM here.
Also see Data Governance.
Location data
Data about locations. Solutions that add location data management to the mix, such as Location Master Data Management, are on the rise. Effectively linking location data to other master data such as product data, supplier data, asset data or customer data can give you a more complete picture and enhance processes and customer experiences.
Matching
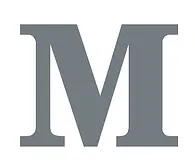
(and linking and merging). Key functionalities in a Customer Master Data Management solution with the purpose of identifying and handling duplicates to achieve a Golden Record. The matching algorithm constantly analyzes or matches the source records to determine which represent the same individual or organization. While the linking functionality persists all the source records and link them to the Golden Record, the merging functionality selects a survivor and non-survivor. The Golden Record is based only on the survivor. The non-survivor is deleted from the system.
Also see Golden Record.
Multidomain
A multidomain master data management solution masters the data of several enterprise domains, such as product and supplier domain, or customer and product domain or any combination handling more than one domain.
Also see Domain.
Metadata management
The management of data about data. Metadata Management helps an organization understand the what, where, why, when and how of its data: where is it coming from and what meaning does it have? Key functionalities of Metadata Management solutions are metadata capture and storage, metadata integration and publication as well as metadata management and governance. While Metadata Management and Master Data Management systems intersect, they provide two different frameworks for solving data problems such as data quality and data governance.
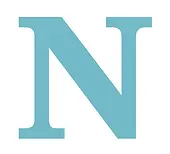
New Product Development - NPD
A discipline in Product Lifecycle Management (PLM) that aims to support the management of introducing a new product line or assortment, from idea to launch, including its ideation, research, creation, testing, updating and marketing.
Omnichannel
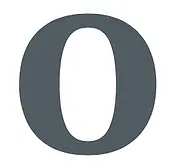
A term mostly used in retail to describe the creation of integrated, seamless customer experiences across all customer touchpoints. If you offer an omnichannel customer experience, your customers will meet the same service, offers, product information and more, no matter where they interact with your brand (e.g., in-store, on social media, via email, customer service, etc.). The term stems from the Latin word omni, meaning everything or everywhere, and it has surpassed similar terms such as multi-channel and cross-channel that do not necessarily comprise all channels.
Party data
In relation to Master Data Management, party data is understood in two different ways. First of all, party data can mean data defined by its source. You will typically hear about first, second and third-party data. First-party data being your own data, second-party data being someone else’s first-party data handed over to you, while third-party data is collected by someone with no relation to you—and probably sold to you. However, when talking about party data management, party data refers to master data typically about individuals and organizations with relation to, for example, customer master data. A party can in this context be understood as an attorney or husband of a customer that plays a role in a customer transaction, and party data is then data referring to these parties. Party data management can be part of an MDM setup, and these relations can be organized using hierarchy management.
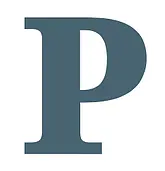
Learn more about party data here.
Also see Hierarchy.
Personally Identifiable Information - PII
In Europe often just referred to as personal information. PII is sensitive information that identifies a person, directly (on its own) or indirectly (in combination). Examples of direct PII include name, address, phone number, email address and passport number, while examples of indirect PII include a combination (e.g., workplace and job title or maiden name in combination with date and place of birth).
Product Information Management - PIM
Today sometimes also referred to as Product MDM, Product Data Management (PDM) or Master Data Management for products. No matter the naming, PIM refers to a set of processes used to centrally manage and evaluate, identify, store, share and distribute product data or information about products. PIM is enabled with the implementation of PIM or Product Master Data Management software.
Product Lifecycle Management - PLM
The process of managing the entire lifecycle of a product from ideation, through design, product development, sourcing and selling. The backbone of PLM is a business system that can efficiently handle the product information full-circle, and significantly increase time to market through streamlined processes and collaboration. That can be a standalone PLM tool or part of a comprehensive MDM platform.
Platform
A comprehensive technology used as a base upon which other applications, processes or technologies are developed. An example of a software platform is an MDM platform.
Profiling
Data profiling is a technique used to examine data from an existing information source, such as a database, to determine its accuracy and completeness and share those findings through statistics or informative summaries. Conducting a thorough data profiling assessment in the beginning of a Master Data Management implementation is recognized as a vital first step toward gaining control over organizational data as it helps identify and address potential data issues, enabling architects to design a better solution and reduce project risk.
Quality
As in data quality, also sometimes just shortened into DQ. An undeniable part of any MDM vendor’s vocabulary as a high level of data quality is what a Master Data Management solution is constantly seeking to achieve and maintain. Data quality can be defined as a given data set’s ability to serve its intended purpose. In other words, if you have data quality, your data is capable of delivering the insight you require. Data quality is characterized by, for example, data accuracy, validity, reliability, completeness, granularity, consistency and availability.
Reference data
Data that define values relevant to cross-functional organizational transactions. Reference data management aims to effectively define data fields, such as units of measurements, fixed conversion rates and calendar structures, to "translate" these values into a common language in order to categorize data in a consistent way and secure data quality. Reference Data Management (RDM) systems can be the solution for some organizations, while others manage reference data as part of a comprehensive Master Data Management setup.
Return on Investment - ROI
Return on investment (ROI) is a measure used to evaluate the profitability of an investment. By calculating the ROI, we are trying to directly measure the amount of return on a certain investment relative to its cost. The ROI is a popular metric used to measure the performance of an investment. Estimate the ROI of your master data management project with our ROI calculator.
Software as a Service - SaaS
A software licensing and delivery model in which software is licensed on a subscription basis and is centrally hosted. SaaS is on the rise, due to change in consumer behavior and based on the higher demand for a more flat-rate pricing model, since these solutions are typically paid on a monthly or quarterly basis. SaaS is typically used in cloud MDM, for instance.
Also see Cloud.
Supply Chain Management - SCM
The management of material and information flow in an organization—everything from product development, sourcing, production and logistics, as well as the information systems—to provide the highest degree of customer satisfaction, on time and at the lowest possible cost. A PLM solution or PLM MDM solution can be a critical factor for driving effective supply chain management.
Stock Keeping Unit - SKU
A SKU represents an individual item, product or service manifested in a code, uniquely identifying that item, product or service. SKU codes are used in business to track inventory. It’s often a machine-readable bar code, providing an additional layer of uniqueness and identification.
Stack
The collection of software or technology that forms an organization’s operational infrastructure. The term stack is used in reference to software (software stack), technology (technology stack) or simply solution (solution stack) and refers to the underlying systems that make your business run smoothly. For instance, an MDM solution can—in combination with other solutions—be a crucial part of your software stack.
Stewardship
Data stewardship is the management and oversight of an organization's data assets to help provide business users with high-quality data that is easily accessible in a consistent manner. Data stewards will often be the ones in an organization responsible for the day-to-day data governance.
Strategy
As with all major business initiatives, MDM needs a thorough, coherent, well-communicated business strategy in order to be as successful as possible.
Supplier data
Data about suppliers. One of the domains on which MDM can be beneficial. May be included in an MDM setup in combination with other domains, such as product data.
Also, see Domain.
Learn more about supplier data here.
Synchronization
The operation or activity of two or more things at the same time or rate. Applied to data management, data synchronization is the process of establishing data consistency from one endpoint to another and continuously harmonizing the data over time. MDM can be the key enabler for global or local data synchronization.
Training
No, not the type that goes on in a gym. Employee training, that is. MDM is not just about software. It’s about the people using the software, hence they need to know how to use it best in order to maximize the Return on Investment (ROI). MDM users will have to receive training from either the MDM vendor, consultants or from your employees who already have experience with the solution.
User Interface - UI
The part of the machine that handles the human–machine interaction. In an MDM solution—and in all other software solutions—users have an "entrance," an interface from where they are interacting with and operating the solution. As is the case for all UIs, the UI in an MDM solution needs to be user-friendly and intuitive.
Vendor
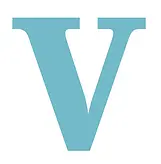
There are many Master Data Management vendors on the market. How do you choose the right one? It all depends on your business needs, as each vendor is often specialized in some areas of MDM more than others. However, there are some things you generally should be aware of, such as scalability (Is the system expandable in order to grow with your business?), proven success (Does the vendor have solid references confirming the business value?) and integration (Does the solution integrate with the systems you need it to?).
Warehouse
A data warehouse—or EDW (Enterprise Data Warehouse)—is a central repository for corporate information and data derived from operational systems and external data sources, used to generate analytics and insight. In contrast to the data lake, a data warehouse stores vast amounts of typically structured data that is predefined before entering the data warehouse. The data warehouse is not a replacement for Master Data Management, as MDM can support the EDW by feeding reliable, high-quality data into the system. Once the data leaves the warehouse, it is often used to fuel Business Intelligence.
Also see Lake and BI.
Workflow automation
An essential functionality in an MDM solution is the ability to set up workflows, a series of automated actions for steps in a business process. Preconfigured workflows in an MDM solution generate tasks, which are presented to the relevant business users. For instance, a workflow automation is able to notify the data steward of data errors and guide him through fixing the problem.
Also, see Business Rules.
Yottabyte
Largest data storage unit (i.e., 1,000,000,000,000,000,000,000,000 bytes). No Master Data Management solution, or any other data storage solution, can handle this amount yet. But, scalability should be a considerable factor for which MDM solution you choose.
ZZZZZ…
With a Master Data Management solution placed at the heart of your organization you get to sleep well at night, knowing your data processes are supported and your information can be trusted.
Want this glossary to-go? Download it and keep it as your go-to master data management dictionary.
Request a free product demo or estimate the ROI of your master data management initiative »